Objective: This study evaluated the performance of a deep-learning-based model that predicted cooking loss in the semispinalis capitis (SC) muscle of pork butts using hyperspectral images captured 24 h postmortem.
Methods and results: Principal component regression (PCR) and partial least squares regression (PLSR) models for predicting cooking loss in SC muscle showed higher R2 values with multiplicative signal correction, while the first derivative resulted in a lower root mean square error (RMSE). The deep learning-based model outperformed the PCR and PLSR models. The classification accuracy of the models for cooking loss grade classification decreased as the number of grades increased, with the models with three grades achieving the highest classification accuracy. The deep learning model exhibited the highest classification accuracy (0.82). Cooking loss in the SC muscle was visualized using a deep learning model. The pH and cooking loss of the SC muscle were significantly correlated with the cooking loss of pork butt slices (−0.54 and 0.69, respectively).
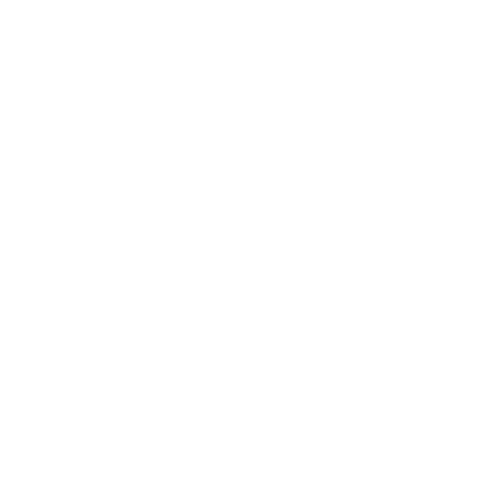
Conclusion: Therefore, a deep learning model using hyperspectral images can predict the cooking loss grade of SC muscle. This suggests that nondestructive prediction of the quality properties of pork butts can be achieved using hyperspectral images obtained from the SC muscle.
Kyung Jo, Seonmin Lee, Seul-Ki-Chan Jeong, Hyeun Bum Kim, Pil Nam Seong, Samooel Jung, Dae-Hyun Lee, Cooking loss estimation of semispinalis capitis muscle of pork butt using a deep neural network on hyperspectral data, Meat Science, Volume 222, 2025, 109754, ISSN 0309-1740. doi: 10.1016/j.meatsci.2025.109754